Robot Doctors, Who’s a Fan of Them?
Speaker 1 (00:00):
Liberty, you and I have talked about this a lot. There's a lot of mixed opinions on artificial intelligence. Some people think it's the future. Some people are worried AI's going to take over and replace humans, jobs, the world. Other people think it might be a little overblown.
Liberty (00:14):
I know. You think it's going to be Ready Player One, or maybe you hope it's going to be Ready Player One. But the other place we're seeing this conversation really explode is in healthcare, where AI is being used more and more. And some people are saying that AI will make healthcare better, but others are pretty scared that AI could cause harm when it comes to their own health.
Speaker 1 (00:35):
Do you think we're going to end up with AI doctors? I don't know. I'd probably let one operate on me, but I'm not sure society is. Do we think this is a misconception that there's going to be an AI doctor? What do we think the future of healthcare AI is going to really look like?
Liberty (00:49):
I think before we define the future of AI, we all have to be on the same page of what it means when we say quote "AI in healthcare." When we talk about AI in healthcare, we're talking about machine learning. So, let's say we want to create a program that detects lung cancer in patients' CT scans. This is how AI would learn to detect something like that. Basically, a large data set is collected of patients who were previously diagnosed, and a human hand labels which scans have lung cancer and which don't. Then this data, with the human label, is fed into an algorithm where the program would analyze the difference between the two groups, lung cancer and non-lung cancer, to determine its own meaning of what lung cancer on a CT scan looks like, so it can identify on new unhuman-labeled cases. So it's interesting because in this case and in future cases, the AI learns based on the data sets the humans collect and label, but the machine really decides for itself what to look for and how to identify something peculiar in a scan.
Speaker 1 (01:56):
That's just an interesting example of AI in healthcare. We want to explore a little bit more, realistically, how much can AI really influence decisions made by practitioners going forward? So for that, we're going to ask Niels Olson. Niels is a board-certified pathologist and chief medical officer at the United States Defense Innovation Unit.
Liberty (02:16):
Niels, I want to understand what AI and healthcare actually looks like. Are we going to get to the point where a doctor doesn't need to go read the X-ray because there's a machine that's read it, and it says the patient has cancer? Is this where we're headed? We want to know what is data's actual role in healthcare?
Niels Olson (02:36):
For pathology, one of the systems it takes, it evaluates the prostate cancer. But it only does it after the pathologist has already made a decision, but before they sign it out. So, it doesn't influence their decision. But if they find cancer, it doesn't adversely affect them finding the cancer. But if, in the rare case that they might have missed something, it'll backstop them and say, "Hey, you should look over here before you sign this thing out."
(03:01):
And then for radiology, there's another somewhat more assertive effort to organize the work list. So as soon as the X-rays come in, the radiologist is getting a list of these things. And they're just coming in in order, time order. So these AI systems can evaluate them while the radiologist is working their way down from the top, and it can look at them very quickly and say, "Hey, this thing looks bad," and it will quietly move that to the top. So, you've got fresher eyes earlier in the day looking at this stuff and getting an answer to the bad things faster.
(03:40):
Now, implicitly, the normals should float to the bottom, and so you'll get to the normals later in the day. What you want to be careful of in that case is making sure that there aren't any bad things in the normals. But generally, it's like Christmas lights. If you're looking for houses with no Christmas lights, the one that has Christmas lights stands out.
Liberty (04:04):
Based on what Niels told us, algorithms can assist doctors in making decisions. But the real question is, are we actually better off using these algorithms?
Speaker 1 (04:14):
One could argue we're better off, with the example the COVID-19 vaccine. When developing any kind of vaccine, clinical trials are the golden ticket to FDA approval. Every vaccine needs to undergo thousands of trials to help protect the patient's safety and increase their ability to fight the virus variants. Before COVID-19, the quickest vaccine to be developed was the mumps vaccine, record-breaking, four-year development period. However, the COVID vaccine was created just under a year, a fact that caused a lot of people to question its validity and safety.
(04:45):
But the thing is, it developed so quickly because of the help from artificial intelligence. Researchers used AI to run trials against different variants. AI was able to change components, tests, and record results for trials faster than any human could. AI also created useful data sets for scientists to figure out underlying causes of successes and failures. So with the help of AI, we were really able to rapidly create a vaccine that saved millions of lives.
Liberty (05:09):
Sure, but this is vaccine development. We have to look at all of the other aspects of healthcare, like detecting and treating cancer, chronic illnesses. And so, for these questions, we decided to bring in Caroline Uhler. Caroline is the Doherty Professor in the Department of Electrical Engineering and Computer Science and the Institute for Data Systems and Society at MIT. She's an electic member of the International Statistical Institute, and she is the recipient of an NSF Career Award.
(05:39):
Caroline, it seems like AI can play a really useful role in healthcare. We saw it with the COVID vaccine development. And we've seen how it can, for example, detect cancer in X-rays. But sometimes more technology, more data, doesn't necessarily mean better care, better outcomes. So, is using an algorithm actually better than the people that we have on the ground?
Speaker 4 (06:03):
Of course, the question is what does better mean? In this case here, what you would like is that you might be able to detect it earlier, right? This is really important here. And I think this is something that has been observed with current methods. Also, some exactly developed by Regina Barzilay at MIT. That they were really able to show that they could detect, in particular in breast cancer, they could actually detect it much earlier than a pathologist could currently detect it. So yeah, an algorithm is able to see many, many more examples than a human ever is, and so might be able to pick up things that are not so evident yet.
Speaker 1 (06:39):
So what are the potential problems with having AI detect and predict people's health conditions?
Speaker 4 (06:44):
I think the questions that we generally have are questions about generalization; whether you are really picking up... And that's why I also work a lot on causality. So, whether what the machine is actually picking up are causal features, and will then generalize also to other settings. Let's just stick to the breast cancer example. You have trained your machine in one particular hospital setting where the images have been taken by a particular person with a particular machine. So now, of course, you want it to then be generalizable to other hospital settings where you might be taking images with slightly different machines and also by other people. And so that your algorithms are not just too specialized to the particular application area that now you're looking at and will generalize also across. And in particular here also, you're going to train it on data from a particular group of people.
(07:36):
And so then you definitely also want it to be generalizable across different populations of people. Because this one particular hospital might be sitting in one particular place. And so, these are difficulties that we currently face and that it's really important to be able to analyze better when these different algorithms do generalize well and when they actually don't generalize well.
(07:59):
And I think here an example is where we are often using data from, say, the UK Biobank, which is of course predominantly European white. And so then it is really important that we actually test and understand in which settings these particular conclusions that we find in this particular dataset will generalize also much more broadly.
Liberty (08:22):
Starting with a non-representative sample or potentially biased sample and then trying to generalize that information to the whole population gives us a problem that people are really starting to recognize in the mainstream news. And that's that machines make biased decisions even when they weren't taught to do so.
Speaker 1 (08:41):
Recently, doctors have been using AI to read chest X-rays. Historically, chest X-rays have never been able to tell the race of a patient. But when initially testing the AI to read chest x-rays, doctors noticed that the AI could, with 90% accuracy, tell the self-reported race of the patient. So to be clear, the problem here isn't the fact that the race of a patient can be determined by their X-ray. The problem is that AI systems tend to diagnose with the patient's race being a factor. And often, the chest X-ray is more likely to neglect a sign of illness in Black and female patients. Scientists haven't been able to determine how medical X-rays are able to tell the patient's race, and they're finding that it might be incredibly difficult to create an algorithm that does not have any racial bias.
Liberty (09:24):
This is an example of machines making biased decisions. But there's another problem. And that's that the machines learn or can learn on biased data. There was this algorithm from a company called Optum, and the algorithm predicted which patients would benefit from extra medical care, with the goal of preventing these people from getting to the point where they needed to go to the hospital.
(09:48):
But the problem was that this algorithm ranked patients based upon health costs, assuming that the more someone paid in medical costs, the more medical care they must need. So, you can start to see what the potential problems with this are. And because of health disparities in the US, African American patients incur about $1,800 less in medical costs per year than the equivalent white person. So with this data, the algorithm ranked much sicker African American patients as equally in need of extra care as much healthier white patients. I think what that means is it's so important to note that the data was not intentionally created to be biased. The data didn't even know if the patient was white or African American. But the existing health disparities were taken into account, which then caused this machine to learn on biased data and give unfair advantages based upon your race.
Speaker 1 (10:44):
So, at the end of the day, these problems can have huge consequences. Most doctors using these algorithms would be unaware of the bias present, which then has the potential of creating a deeper disparity in healthcare.
Liberty (10:56):
So we wanted to go back to Niels Olson to ask, "What does it take to fix the bias in data?" We've seen that there's enormous bias in the data. And we have AI systems that will rank much sicker African American patients as equally in need of extra care as much healthier white patients. You see this bias in the data that happens regularly with insurance systems or whatever. So, could that same issue be applied in an AI setting with medical data where you get enormous bias from the data itself that makes the whole system wrong?
Niels Olson (11:29):
Actually, I think this is a place where AI is a solid win because you can measure it. You can measure the bias. I know how much, and I can go hold the algorithm developer accountable. What it does do is put incentive on developing those test sets, so I can do the measurement of how much. And I think that's something that most of these AI conversations miss, is the massive value in developing independent test sets for verification and validation. That independent verification/validation step is critical. And the algorithm developers don't want to talk about that cost. That's really expensive.
Speaker 1 (12:16):
So, it seems like there's some methods and possibility of solving biases and algorithms, but like Niels says, it's expensive.
Liberty (12:23):
And so, I think when we were talking to Caroline, I was really curious how she approaches this problem of bias, and I guess specifically what needs to happen for it to be fixed. Caroline, how do we make industry actually care to fix the problem of biased algorithms. Besides doing the right thing, are there other incentives we can give them?
Speaker 4 (12:46):
A lot of people do want to fix this. And so, there is a lot of really important research going on in this area where again, it's always this counterfactual question of understanding whether if all I changed is race, for example, would the outcome be different? If everything else would be the same, but this is something that I'm changing. And so, this is the counter. And if this is a problem, if this is really the case, then obviously something is wrong in the data set, that there are biases in there in the data set that you don't want to be perpetuated. And so, this is the type of research that we're doing quite a bit of, in terms of really trying to understand these counterfactuals and really trying to understand where, you already said in the story, where is it that it went wrong?
(13:31):
But this required a whole analysis of identifying what is actually these factors that are these causal factors that then lead to these downstream effects that you don't want it to have? And so, I think just this research in this area of really trying to understand what are the causes that then lead to a particular decision that is being made is super important, so that we don't run into this problem of just using some biases that are just in the data set.
Speaker 1 (13:55):
If we can solve these problems with the datasets, and it seems like we can, what are you really excited about in the next 10 years when it comes to using this kind of technology in healthcare?
Speaker 4 (14:04):
Yeah, I really think it is just super exciting times right now where we can really make big advances with machine learning in drug development, in the identification of different types of diseases, but also just in really fundamental understanding of different diseases and the biological mechanisms underlying some of the diseases. So I think it's just a super exciting time, and I think we've only seen really the tip of the iceberg where a lot of just the automation kinds of things have entered into drug development where we are using AI.
Liberty (14:36):
All right. So what do you think is the next big medical breakthrough? Wave your magic wand for a second. Do you think we will have solved anything huge in the next 10 years?
Speaker 4 (14:47):
Yeah, I think for some diseases we've gotten already quite good at really looking at them. But others, we're still far away. Something that I like to say often is that, for example, cancer has been cured many times over in mice, but we're still not very good at curing it in humans. So there is a big step there to be done that we haven't done yet.
(15:07):
And I think the other one is also neurodegenerative diseases. I think, we're very, very far away from really understanding them and having a cure, so that we can not just become old, but actually healthily old. I think healthy aging is really one of the important steps to do. You actually want to become old and really be able to have a great life at that time.
Speaker 1 (15:31):
AI in healthcare is heading in a really revolutionary direction. But are we really ready for this?
Liberty (15:36):
Well, Scott, I asked my students, who are obviously a totally different generation, to talk to people in their circles about how they'd feel with receiving a diagnosis from AI. So they went out and they asked people of many different ethnicities, backgrounds, ages, first about receiving a lung cancer diagnosis, but then about receiving a sleep apnea diagnosis. And they'd preface the questions with the fact that the AI program would be statistically more reliable than the average human doctor.
(16:06):
What was really interesting is that people that didn't have chronic illnesses or frequent doctor visits, aside from the once-a-year checkup, were more likely to say that they would trust the AI program's diagnosis. And this was very especially true regarding the sleep apnea diagnosis because, I would assume, it's a less severe condition. But those that consulted with doctors frequently, people with chronic conditions or medical issues or whatever, they were a lot less likely to side with the AI diagnosis.
Speaker 1 (16:38):
That's logical to me because I would think people with severe medical issues would want a second opinion, whether it's AI or just another doctor.
Liberty (16:45):
Well, sure. And what's super interesting is another factor people brought up was that it was dependent on how the AI diagnosis was delivered. One of my students asked her mother if she would trust an algorithm to deliver a diagnosis to her. And her mom said that she would be skeptical of a major diagnosis unless the information was delivered to her by Baymax, that adorable, little, inflatable robot from Disney's film. I'm sure you've watched this many times, Scott. Big hero six. But my student, she was pretty surprised and asked her mom why. Her mom explained that it would make the algorithm more tangible and would feel more human-adjacent. She still wanted that human touch.
(17:28):
And a lot of the student's peers agreed with her mom, that if they were told via computer that they have lung cancer, they wouldn't trust the AI diagnosis. But if they had a human contact telling them the AI diagnosis, then they'd be a lot more comfortable.
Speaker 1 (17:43):
That poses the question, will we ever get to the point where we fully trust AI to diagnose us or treat us? Will it eventually take the lead? Will it replace a doctor?
Liberty (17:54):
Well, I'm going to fob off the hard question to Niels Olson again because I know he's spent a lot of time really considering if people will ever come to truly trust the data. Niels, I have to ask the pointed question. Do you think AI will get to the point where it replaces doctors to the degree that we'll actually need to fully trust AI and not have a person?
Niels Olson (18:16):
No. These are non-trivial decisions. I'm actually a military officer, and I was a service warfare officer before going into medicine. And we had autonomous weapons systems in the eighties. The Aegis cruiser system could shoot a missile without anybody ever making a decision. Just set it on auto, and it will start shooting things if they need to be shot. Right? No one ever trusted it because starting wars is a big deal.
Speaker 1 (18:45):
That's the understatement of the conversation.
Niels Olson (18:48):
I don't think anyone is ready to let these things make decisions like chemotherapy. If I say somebody's got cancer, then that gives an oncologist not only permission, but an obligation to inject them with chemicals that could kill them. Chemotherapy is basically just enough poison to not quite kill you. And that is a big deal. It gives a surgeon not just permission, but an obligation to cut you open. That's kind of a big deal. So, I don't think anybody is ready to do that without human intervention. It would be nice if we could make the workload easier, but we're going to want somebody to sign for that decision.
Speaker 1 (19:31):
You want accountability.
Niels Olson (19:32):
Right. Comes down to accountability. If my Tesla... I drive a Tesla. I've tried putting on it's full auto mode. And yeah, my regular car, I might drive with one hand sometimes. But the Tesla, I have two hands on the wheel all the time.
Liberty (19:46):
Oh, that's interesting. I feel like the point of my Tesla is that I don't have to have my two hands on the wheel all the time.
Speaker 1 (19:52):
I was going to say, it's interesting you bring up the full autonomy thing, like in the Tesla. The argument is, "Look, it's not that the Tesla or these automated systems don't make mistakes. It's just that, statistically speaking, they make less mistakes than humans." But statistically speaking, autonomous cars probably make less mistakes than human drivers. They're not checking their phones and things like that. Is that kind of logic okay to apply to medicine?
(20:18):
To your point, we probably don't want them diagnosing cancer all the time. But if statistically speaking, they're better than a doctor who hasn't slept in three days because they're on call, it's not that it's not going to make mistakes; it's going to make less mistakes. Computers aren't necessarily perfect. They can still make mistakes. But statistically speaking, they can be better at certain jobs than humans are, as long as they're programmed. So when applied to medicine, do you think we'll ever be okay with the trade off and let AI take on more responsibility?
Niels Olson (20:45):
I don't see it happening. We have one project where it's deidentification, where we want to share free text medical records with researchers. And it would be nice if we could share it and be confident that it's deidentified. Okay, we've actually evaluated multiple de-identification systems. The probability that they miss a name is very low, but it's not zero. And so, I'm still going to have to pay somebody, preferably two or three people, to actually look at it before we let it out the door. I'm going to have names that I can identify and sign. This person looked at it, said it's completely clear. And there's consequences if they get it wrong. And that's just de-identification for medical research. There's not really patient harm.
Speaker 1 (21:32):
It's low stakes, is what you're saying.
Niels Olson (21:35):
Right. Relatively low stakes compared to making new chemotherapy drugs.
Liberty (21:41):
I like this concept because I don't care how... I'm a data person. And you could tell me that the computer is 99% accurate and the doctor's 90% accurate. I still want the doctor to sign off on it in the end. I get that. The idea is, in 50 years are we going to feel differently or not? But I get that concept.
Speaker 1 (21:57):
It's interesting you say that. I'm okay with that. And maybe it's maybe I trust the data and the computer so much. But I'm not necessarily sure I'm going to be the prevailing opinion in our lifetime. I think your opinion's going to be the prevailing opinion, is people are just, "The autonomous system you worked on in the eighties worked, but no one wanted to risk starting a war because of it." So do you think in the future AI is going to be a net positive for healthcare, or do you think it's going to be a net negative because of the bias that comes into it and the lack of trust?
Niels Olson (22:25):
I think it's going to be a net benefit. And I'd say anytime you have more evidence, I don't know of any judges or attorneys who are sad to get more evidence. So, as someone making diagnostic decisions on a regular basis, that ability to have more evidence, and you can think of it in this setting of let's say I have a augmented-reality microscope, and it has a prostate cancer algorithm trained by 29 pathologists. I can say, "This thing made this inference, which is a digital file, and that is the aggregate opinion of 29 other pathologists. I disagree with it because of the following." But at least I can compose that sentence where it starts with "I disagree" or "I agree."
Liberty (23:15):
Well, I think this has been certainly one of our most confusing episodes. But I do think that I'm wrapping my mind around the subject a lot more. What about you, Scott?
Speaker 1 (23:25):
Yeah, I think I am too. It's interesting how AI is going to be the next great medical tool, assisting diagnosis and things like that. And we've had some astounding medical victories recently with the COVID vaccine. I think AI's really got some promise for the future.
Liberty (23:39):
Yeah, but to have the chief medical officer of the US Navy's Defense Innovation Unit tell us the idea that people won't trust the data either way is pretty incredible.
Speaker 1 (23:51):
Yeah. Not trusting the data seems to be a pretty common theme as of late. But I don't know that it's really something we've got to worry about. Robot making our healthcare decisions in the near futures; we're not going to take the person out of the loop. AI's going to be here in many ways with assisting in diagnoses and potentially surgeries and things like that. And as always, you just got to be the best advocate for your own healthcare.
Liberty (24:13):
Yeah, I think it's pretty clear that even with AI playing a role, the backbone of our healthcare system will stay as it's always been: people helping people.
Creators and Guests
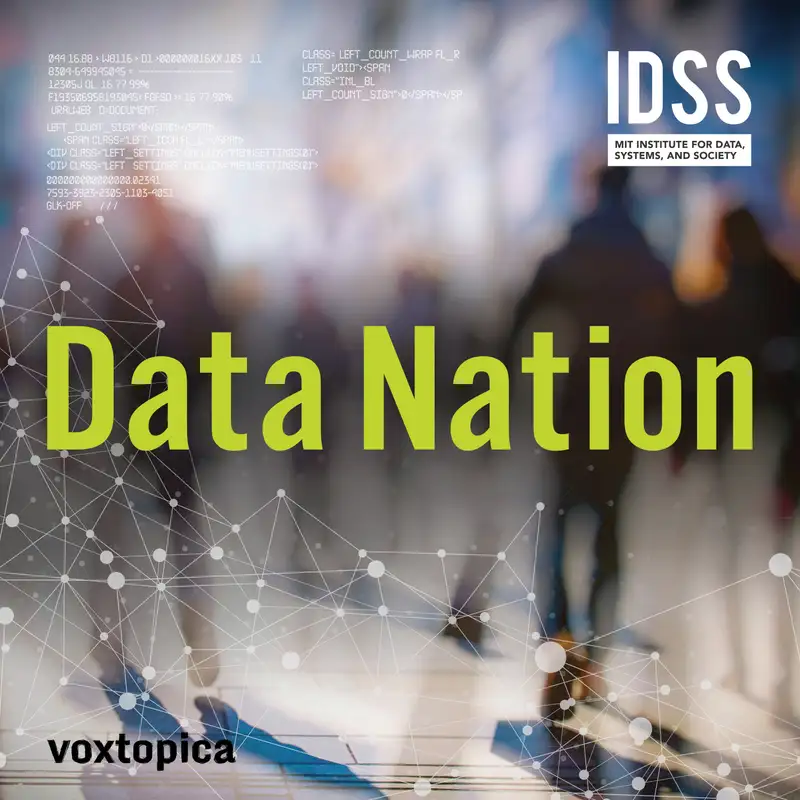