Analytics, Are They Ruining Sports?
Munther (00:04):
Welcome to Data Nation. I'm Munther Dahleh, and I'm the director of the MIT's Institute of Data, Systems, and Society. Today on Data Nation, Liberty and Scott are exploring the impact of data and analytics in sports.
Liberty (00:25):
Everyone has a unique relationship to sports. Some people are rabid fans of their sports team and other people, like me, could care less, but still go to watch events with friends for the social aspect. But whatever your relationship is to sports, sports are really important. Cities local economies benefit from their teams and sports in general are a major aspect of culture. But you should know that sports are shifting. Analytics are really taking over in every sport and people are wondering, is this a good thing? I mean, are analytics really worth the money and the resources? And are they maybe ruining sort of the beauty or pureness of the game?
Scott (01:12):
The concept of analytics and sports really began with the Oakland Athletics. You may have heard of the 2011 film Moneyball, based on the book Moneyball. Moneyball tells the real story of the As and their attempt to use analytics to field a competitive team despite being the second poorest franchise in baseball.
Liberty (01:28):
But okay, don't all teams have an equal shot at adding new or talented players to their roster?
Scott (01:35):
That's not the case at all. So in major league baseball, teams don't have a salary cap, meaning there's no limit to the amount of money they can spend on their players. In 2002, the New York Yankees had the highest payroll in the MLB at 140 million. While the Oakland A's had a payroll of just 40 million. This creates such a huge disparity and a dynamic where smaller markets such as the As train young players in their farm system, invest in the young talent with the hopes of creating talent that can help the team win in the future. When these players do become elite, they start looking for more money though. This creates a bidding war that can only be won by large markets like New York or Boston. The As were basically stuck in this endless cycle of raising promising young talent only to lose them to larger markets.
Liberty (02:17):
Well, so then what do they do?
Scott (02:19):
Basically, they turn to analytics. Oakland A's general manager, Billy Beane with the help of assistant general manager, Paul DePodesta, used analytics to find overlooked and undervalued players to field a competitive team on a low budget.
Liberty (02:32):
Okay, well, did it work?
Scott (02:34):
It did, except for, they didn't win the World Series, but they did win 103 games and tied for best in the MLB. Shocked the world of baseball with a 20 game win streak that still hasn't been surpassed. But Liberty, it was bigger than this. This signaled a huge change in the guard of professional sports. One in which teams used advanced statistics to make in-game decisions, determined players' values, all the things they hadn't been using to win world series for the past 60 years, they were using these fancy new mathematical terms to get an edge.
Liberty (03:05):
Today it seems like nearly every action and inaction of each player on the playing field can be quantified and measured. And teams use these measurements to get the upper hand on their opponent. And I'm curious what this means for sports. I mean, it seems like a positive to use analytics to build a strong team, but is it a good direction? Does this tracking of data really ruin the game?
Scott (03:31):
The person who would know the answer to this question is Brian Bilello. Brian is the president of the New England Revolution. Brian has worked closely with the Kraft family to represent the Revolution in league matters. And during this time, the Revolution had been to four major league soccer cups.
So Brian, I was just talking with Liberty about Moneyball and how analytics are playing a major role in sports. We know that analytics can really affect the outcome of the game, but it's more than that. How are advanced data collection and analytics affecting business decisions in sports?
Brian (04:01):
It's an interesting question because what you have to really do is understand for whether it's a league or a team, what's your goal? What are you trying to accomplish? And then you can employ the analytics to reach whatever goal you want. So if your goal is to win as many games as possible, well, the analytics can lead you towards paths that will help you achieve that. But if you're in a baseball team and you're like, we just want to score as many runs as possible. We don't even care if we win games. The analytics would probably tell you not to buy any pitching and to buy more hitters. So I think you have to set that goal for yourself and then the analytics can help you achieve whatever goal you want. Most teams have some level of budgeting that they're working through. And so if you can use analytics to more effectively make yourself competitive, then you can do better than your competition.
So I think if you can find a competitive advantage to analytics, ultimately that does help you on the budget because you're finding... In theory, the whole Moneyball thing was just, they realize certain qualities were more important than they were valued in the marketplace. So they could put together a better team for less money. Because what was actually being valued was not the right metrics. I think there's a lot more to go and it varies a little bit by sport. So when you look at something like soccer, I think there's a long way to go to really understand situations where really we're using visual spatial data right now, but it's early days in doing that. So I think there's a lot on the tactical data that we still have to do. I think the physical data across sports, so physical performance, health, we call it performance in soccer, but really physical, running, endurance, those types of traits. I think all sports have some work that we still can do there to help you understand athlete performance from that perspective on top of the tactical.
And then finally really important in soccer and again, not shared with a lot of the other sports is scouting data. And so when I say scouting data, it's really, how do you take player performance data from a different league team or even age bracket, and then use that to predict performance if that player was on your team? So if I'm looking at 15, 16, 17 year old kids in our academy, can I use that data to project what their ceiling might be with our first team? If I'm scouting a player in Belgium and I know how they perform in the Belgium league, how do I translate that to a likely performance for my team? And so there's so much international movement from players, from club to club and maybe going from a club that's not as good to a club that's better, to a club that plays more defensively, to a club that's more attack minded. It's not as simple as baseball where you say, well, if the players are hitting 270 and has this OBP and this whatever, and they're playing for the Baltimore Orioles, if they get traded to the Red Sox, they're facing basically the same pitchers and they're the same batter. So yeah, maybe there's some ballpark stuff in terms of the dimensions and stuff like that. But generally speaking, it's the same situation.
Scott (07:05):
So a lot of people are wondering if we're going to start seeing computers and algorithms being used on the sideline in real time. One might argue that the math and science is there, but is the game ready?
Brian (07:14):
I do think it's always going to vary to a degree by sport. I'm not sure that's not happening today in baseball. So I'm not saying every team across the league, but again, I think baseball is the easiest one analytically to make those decisions.
Scott (07:33):
And it's slow enough.
Brian (07:35):
Yeah. It's all situational, right?
Scott (07:37):
It's not an action game. It's a suspense game. You can have a computer interject.
Brian (07:41):
Yeah. I think the NFL can do that to a degree. Because again, you have got to play. You've got a down and distance. You've got a score. You've got a time. You've got all these fixed variables that...
Scott (07:52):
Can be interpreted.
Brian (07:53):
Can be interpreted. I do think there's a lot of improvisation that happens on the field though in terms of calling audibles and seeing what you see. And ultimately if it's a passing play, the quarterback has to step back and read the situation and make a decision as to what to do. So I don't think it becomes totally like that. Soccer is really tricky because we don't have timeouts. We have one stoppage, which is halftime. You can't make subs on the fly. So you can't say I want to change this match up because of this. So I think that one is going to be a little bit trickier to make in-game decisions because the players are really making those decisions. We're looking at things like set pieces and other areas where maybe we can gain some insights that will help you.
Scott (08:31):
So do you think there are significant amount of organizations who have different opinions about how analytics should be used in sports? Are there different schools of thought around this or is everyone on the data analytics train and ready to incorporate it as much as possible?
Brian (08:43):
It's not the latter. I mean there's varying views on this and use of. I take it up a level, just societal in terms of data and analytics. As human beings, I think a lot of people don't trust what they don't understand. We're trying to explain to a coach, a scout or somebody that this player may not be as good as you think they are. And the reason is because of all this data that we have someone who's got a mathematics degree and you're lead series of equations is why we think this player may not be as good. That soccer person in our case is never going to understand the analytics.
Scott (09:21):
Here's my MLS championship. Show me your math again.
Brian (09:25):
So I do think the uphill battle you have anytime you're trying to explain an answer that someone doesn't intuitively get, but also doesn't have the skillset to understand the math, that's really hard. If you're a sporting director and you keep putting together bad teams, no one's going to hire you to be a sporting director anymore. So your very career is based on these decisions and you making decision based on something that you fundamentally don't understand. I think that's really hard to ask people to do. And so what you try to really work on when you're developing new analytics is helping them understand basically where it comes from and the best tool you have in that case is say, okay, so for instance, if we have something like trying to show expected goals versus goals and why it's important, well, let's just show you the players in our league and rank them all by expected goals.
And what you'll see is the top players are up there. And then you talk about the guys that you think are good that don't rate well there, or the ones that you don't think are good that do rate well there. And that's where the interesting conversations take place. And then you can help them start to get the insights themselves in a different way because some of that data, once you see it and you put a name to it or you show a possession type and say, this is why this is dangerous, they'll start to understand, okay, I get it now. And then as they gain trust in certain analytics, they become part of your process and part of your culture.
Scott (10:49):
So it doesn't seem like analytics are going to ruin the game just yet, but it does seem like it's difficult getting everyone on board with using data to their advantage. The thing is, it's not just sports teams are wanting to put this data to use.
Liberty (11:02):
The world of sports analytics is actually a really big money industry. It's bigger than the US alcohol industry and really only growing. In 2018, the US Supreme Court overruled the Professional Amateur Sports Protection Act of '92 revoking the federal ban on sports betting. So this opened up new avenues in the world of gambling where entertainment companies could now offer many different ways for people to gamble. Today, in the 30 states where sports betting is legal, 18.2 million Americans gamble on their favorite sports, creating an industry that generated over $52 billion in revenue in 2021. This is equivalent to if every single American bet $155 on sports each year.
Scott (11:53):
That's huge. I mean, in the same way, sports executives use analytics to make endgame decisions to improve their chance of winning, sports gamblers, like myself, use these fancy analytics and stats to improve their chances of winning a bet.
Liberty (12:06):
How much money have you lost?
Scott (12:08):
It's not about how much you lost. It's about how much fun you've had watching.
So what does this all mean for the fan that gambles? How can people actually use this data? And what is the future of analytic sports betting?
Liberty (12:20):
To find this out we're talking with professor Anette Peko Hosoi. Professor Hosoi is the sports data and technology expert at MIT. She is the Pappalardo Professor of Mechanical Engineering at MIT.
Scott (12:33):
Professor Hosoi. You are an expert on sports data and we were just talking with Brian Bilello, the president of the New England Revolution about how sports teams are actually using analytics today and how advanced analytics really are. Based on your experience, where is data analytics in sports headed?
Professor Hosoi (12:50):
I think one of the things that we're really fortunate in now is that we're just tracking so many things that we couldn't track before. If you think about the early 1900s in baseball, and by the way, at MLB, you can get play by plays for games that were played in 1912 in baseball. So the data goes way, way back. What's different now is that we can start to automatically track things. So you don't have to write everything down by hand. So for example, in the NBA, there's a company called Second Spectrum that is tracking all of the athlete movements. So you get X and Y position of every player in the NBA and every game at 25 Hertz. So you really get a more precise level of every action that an athlete takes, which means that you have to develop more and more sophisticated data analysis tools in order to take in this enormous amount of very heterogeneous data.
Liberty (13:38):
When it comes to sports data, what else have you found in your research? What other information can it tell us?
Professor Hosoi (13:46):
So, one of the things that I'm really interested in in the sports data is how you can use that to evaluate human decision making. So one of the nice things about sports is that in sports, every decision you make manifests as a physical action or a physical inaction. And so somehow something about the quality of a player's decision making must be hidden in that data. So for example, one of the things that we've looked at is we've looked at NBA tracking data, and imagine the following scenario. So imagine I know the positions and the velocities of every player on the court. So one thing I can do is I can freeze that Tableau right before a player takes a shot. So I now know the positions and velocities right before the guy takes a shot. And then I can just measure how often does the shot go in when you're in that configuration?
So I can learn the probabilities that a shot is going to be successful based on the positions and velocities of the players. I can also learn the probabilities that if instead of taking the shot, he had made a pass to one of his fellow teammates and I can learn the probability that that pass would've been successful. And then I also know, since I know the probabilities of the shots, I know if the person he passed to takes a shot, I know the probability as to whether that shot was going to be successful. So now I can do the comparison. So imagine the following; so I look at every possession, I freeze the possession right before the guy takes a shot, and now I just do the following computation. I ask, what is the probability that his shot goes in versus what is the probability that if he passed to one of his teammates that their shot would go in? And I can ask, which of those is the higher probability? And from that, determine whether or not he made a good decision. Did he take the highest probability score on the court?
Liberty (15:21):
Is it true that these analytics are actually going to help my ability to bet? Or is it really that sports betting and especially for the everyday person is more based on luck and the algorithms don't actually matter in the long run?
Professor Hosoi (15:35):
Yeah. This is a terrific question. And I think we're going to see an enormous amount of evolution along that front, because like you said, the rules around betting have now changed. When I'm playing fantasy sports, am I flipping coins or am I actually making these decisions based on some kind of statistical inference, which gives me an edge in the game? And what we found is that in fantasy sports, the outcome is skill based and it's typically skill based to the same extent that the counterpart real sport is skill based. So in the following sense, any activity that you do is going to have some elements of skill and some elements of luck. The fact that you made it to work without being hit by a car is mostly skill about your driving, but you also got lucky that nobody rear ended you. So every activity you have is going to have some balance of skill and luck. And so when you're talking about whether something is an outcome of skill versus luck, you're really asking, where does it sit on this spectrum? And in sports, when you're betting on sports, having those statistical algorithms and having that statistical knowledge makes a difference.
Scott (16:35):
So some games are more skill based and some games are more luck based. How does that impact the outcomes of games and sports in general?
Professor Hosoi (16:42):
First, let me be clear about what we mean by skill and luck. So I am not saying that a hockey player is more skilled than a basketball player or vice versa. In comparing skill and luck, what you are asking is, do the rules of the game reward skill? So meaning, do the most skillful players or the most skillful teams come out at the top? So basically if there's anything that makes you in some way better at the game, then that counts as a skilled outcome, because it means that the outcome is slightly more predetermined. For example, in basketball, being tall counts as a skill. So the question is, so if I look at the end of the season, if I look at the rankings of the players or the rankings of the teams, does that ranking reflect the quality of the team? And if the game I'm playing is flipping coins, then it's not reflecting any quality. If the game I'm playing is chess, then that ranking is pretty good. Because the outcome of chess is largely determined by skill.
Liberty (17:37):
All right. So before we wrap this up Peko, if we, or me, is going to go start a fantasy league after this conversation, what would be your best tip or trick for betting on games?
Professor Hosoi (17:54):
So if you're, for example, playing fantasy sports with your family or something. So I should say this, I do play fantasy sports and I will tell you that when my nieces were eight years old, I crushed them in fantasy football. It was great.
Liberty (18:08):
It's a real claim to fame. You crushed those eight year old girls.
Professor Hosoi (18:10):
Exactly. That's right.
Liberty (18:12):
I mean, I know Scott likes crushing 13 year old boys, although when they're 14, he can't when they are playing World of Warcraft or whatever it is.
Professor Hosoi (18:20):
Exactly, exactly. So if you are planning on just destroying your 13 year old nieces and nephews in this, then I recommend doing something where you are actually closer to the luck end of the spectrum. And honestly that actually makes it a more fun game for the family because then everybody has a chance to win. And so if you're doing it for entertainment, then something like football, where there is a lot of randomness, is great. If you're doing this to make money, then I would recommend something that is more deterministic, like basketball. And so again, just because there's so much averaging that happens over one game, if you do the statistics well, then the probability of ending up on top versus people who are not doing the statistics is higher. So I would say if you're trying to make money, go for something more deterministic. If you're trying to have fun with your family, go for something random.
Liberty (19:13):
Sports analytics are clearly here to stay. Teams can use these analytics to make more competitive teams. Clubs can make more lucrative decisions and fans, like us, can use the data to put more money in their pockets.
Scott (19:28):
The analytics are only getting more sophisticated. As of right now, I think we can agree it's not ruining sports, but it does change the game for sports betting. So if you're looking to win money and beat your friends, take Professor Hosoi's advice, bet on basketball. Just maybe don't bet a whole lot.
Liberty (19:48):
Thanks so much for listening to this episode of Data Nation. This podcast is brought to you by MIT's Institute for Data, Systems, and Society. And if you want to learn more about what IDSS does, please follow us at MIT IDSS on Twitter or visit our website idss.mit.edu.
Creators and Guests
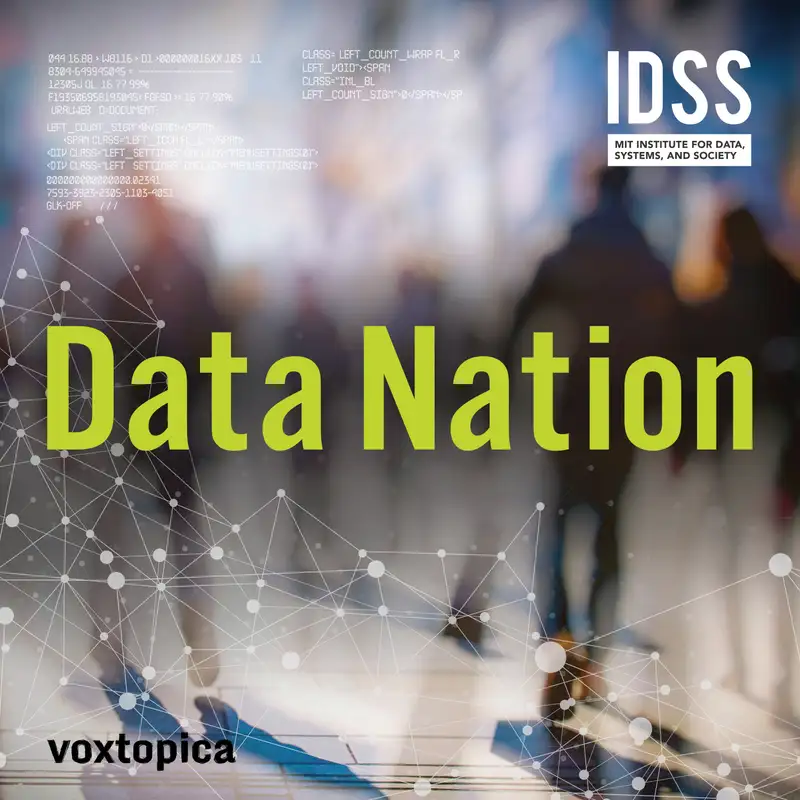